Agriculture is the backbone of India’s economy, employing millions of people and feeding its vast population. In recent years, technological advancements, particularly in artificial intelligence (AI) and machine learning (ML), have revolutionized various industries, including agriculture. This article explores the potential of AI and ML in Indian agriculture, the challenges they aim to address, and the transformative impact they can have on crop yield, resource management, and sustainable farming practices.
1. The Need for Technological Advancements in Indian Agriculture
Indian agriculture faces various challenges, necessitating the integration of advanced technologies.
- a. Population Growth: With a growing population, there is an increasing demand for food production.
- b. Climate Change: Climate change poses risks of extreme weather events and uncertain rainfall patterns.
- c. Resource Scarcity: Efficient resource management becomes crucial amid water scarcity and depleting arable land.
2. Understanding AI and Machine Learning in Agriculture
AI and ML technologies involve the use of data and algorithms to improve decision-making and optimize processes.
- a. AI in Agriculture: AI systems emulate human intelligence, analyzing data and patterns for better agricultural outcomes.
- b. ML in Agriculture: ML algorithms enable machines to learn from data and make predictions without explicit programming.
3. Precision Farming: Optimizing Resource Use
AI and ML enable precision farming, optimizing resource utilization for improved productivity.
- a. Data-Driven Decisions: Sensors, drones, and satellites collect real-time data for better decision-making on irrigation, fertilization, and pest control.
- b. Predictive Analytics: ML algorithms predict crop health, yield, and potential disease outbreaks, aiding preemptive measures.

4. Crop Yield Prediction and Management
AI and ML algorithms empower farmers to predict and manage crop yields effectively.
- a. Yield Estimation: ML models analyze historical data, weather patterns, and crop conditions to predict potential yield.
- b. Crop Management: AI systems provide insights for crop rotation, pest management, and optimal sowing time.
5. Disease Detection and Control
AI and ML technologies play a critical role in disease detection and control.
- a. Image Recognition: AI-powered image recognition identifies diseases, pests, and nutrient deficiencies in crops.
- b. Early Warning Systems: ML algorithms detect early signs of crop diseases, enabling timely action to prevent widespread outbreaks.
6. Weather Forecasting and Risk Mitigation
AI-driven weather forecasting enhances risk mitigation strategies for farmers.
- a. Accurate Predictions: AI models process vast amounts of data for precise weather forecasts and climate predictions.
- b. Climate Risk Analysis: Farmers can plan for extreme weather events based on AI-generated climate risk analysis.
7. AI-Enabled Irrigation Management
AI assists in optimizing water usage through efficient irrigation management.
- a. Soil Moisture Monitoring: AI sensors assess soil moisture levels, reducing water wastage and ensuring appropriate irrigation.
- b. Automated Irrigation Systems: AI-driven automated irrigation systems adjust water supply based on real-time data.
8. Crop Health Monitoring with Drones
Drones equipped with AI and ML capabilities revolutionize crop health monitoring.
- a. Remote Sensing: Drones capture multispectral images, providing valuable insights into crop health and stress levels.
- b. Precision Spraying: AI-driven drones target specific areas for pesticide application, reducing chemical use.
9. AI in Agricultural Robotics
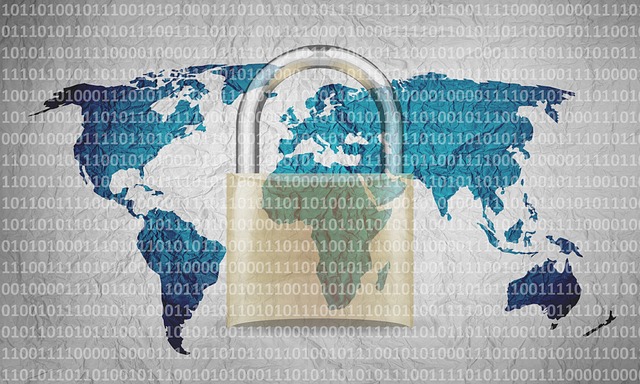
AI integration in agricultural robots enhances automation and labor efficiency.
- a. Harvesting Robots: AI-powered harvesting robots can autonomously harvest crops, reducing manual labor requirements.
- b. Weeding Robots: AI-driven weeding robots identify and remove weeds without harming crops.
10. Addressing Farmer Knowledge Gaps
AI-based agricultural apps and platforms address farmer knowledge gaps.
- a. Information Dissemination: AI platforms deliver vital information, agricultural best practices, and weather updates to farmers.
- b. Advisory Services: AI-driven chatbots offer personalized agricultural advice to farmers.
11. Sustainable Agriculture Practices
AI and ML contribute to sustainable agriculture practices and environmental conservation.
- a. Soil Health Management: AI models monitor soil health and suggest organic farming techniques.
- b. Crop Diversification: AI-guided crop diversification improves soil fertility and reduces the risk of pest outbreaks.
12. The Road Ahead: Policy and Adoption Challenges
For AI and ML to reach their full potential in Indian agriculture, certain challenges must be addressed.
- a. Data Access: Ensuring data access and privacy are crucial for AI and ML adoption.
- b. Training and Awareness: Farmer training and awareness programs are essential for embracing new technologies.
- c. Policy Support: Supportive policies that incentivize AI and ML adoption in agriculture are necessary.
Conclusion
AI and ML have immense potential to transform Indian agriculture, addressing challenges and optimizing resource use for increased productivity and sustainability. From precision farming to disease detection, AI-driven technologies offer solutions that can benefit both farmers and the overall agricultural landscape. By embracing and supporting the integration of AI and ML in agriculture, India can enhance food security, reduce environmental impact, and strengthen its position as a leading agricultural nation. As technology continues to advance, the journey towards a technology-driven and sustainable agricultural future in India is set to accelerate, creating new opportunities and driving positive change for millions of farmers and the entire nation.